It requires a large corpus or body of language from which to learn. Over time, it seems likely that the same improvements in intelligence that we've seen in other areas of AI would be incorporated into physical robots. For example, researchers at CCDS have developed a machine learning algorithm that can detect motion when a patient is undergoing an MRI scan. Through information provided by provider EHR systems, biosensors, watches, smartphones, conversational interfaces and other instrumentation, software can tailor recommendations by comparing patient data to other effective treatment pathways for similar cohorts. The new PMC design is here! . Numerous studies have demonstrated the ability of AI and other analytics tools to predict kidney disease, identify breast cancer, and accurately forecast leukemia remission rates. Clinical decision support tools have been around for a number of years, but many of them have been somewhat standalone solutions and not well-integrated into the clinical point of care devices that people are using, Katherine Andriole, director of research strategy and operations at the MGH & BWH Center for Clinical Data Science (CCDS), told HealthITAnalytics.

Deep learning to predict falls in older adults based on daily-Life trunk accelerometry, Predicting 30-Day readmissions: performance of the LACE index compared with a regression model among general medicine patients in Singapore. However, that often doesn't matter if the patient fails to make the behavioural adjustment necessary, eg losing weight, scheduling a follow-up visit, filling prescriptions or complying with a treatment plan. Machine learning itself will not solve the problems that clinical decision support already has, but it can make certain parts of clinical decision support more effective, said Sendak. Even though, as we have argued, technologies like deep learning are making inroads into the capability to diagnose and categorise images, there are several reasons why radiology jobs, for example, will not disappear soon.29. . What was a little bit surprising was that we don't actually have complete death data, especially for patients who are discharged from the hospital, and this is true of many institutions, Sendak noted. While collecting information, researchers discovered that they were missing come crucial data points.
arrange Who is the right person to share this information with, and when? We can just go ahead and re-scan them rather than go through the process and cost of having them come back in because their imaging wasnt adequate, Andriole explained. Mistakes will undoubtedly be made by AI systems in patient diagnosis and treatment and it may be difficult to establish accountability for them. These NLP-based applications may be useful for simple transactions like refilling prescriptions or making appointments. Electronic health records and the data within them are not necessarily designed for downstream use in algorithms. about navigating our updated article layout. As the saying goes, garbage in, garbage out, said Mark Sendak, MD, population health and data science lead at the Duke Institute for Health Innovation. Surgical robots, initially approved in the USA in 2000, provide superpowers to surgeons, improving their ability to see, create precise and minimally invasive incisions, stitch wounds and so forth.6 Important decisions are still made by human surgeons, however. Physical robots are well known by this point, given that more than 200,000 industrial robots are installed each year around the world. Even with all these advancements, however, the industry still struggles with several foundational problems. As a result, the explanation of the model's outcomes may be very difficult or impossible to interpret. We are likely to encounter many ethical, medical, occupational and technological changes with AI in healthcare. A proven assessment model for evaluating new technology, The Smart Hospital: In-Patient Remote Monitoring, AI and Healthcare: How to Bring Analytics and AI Into the Clinical Setting, How Limitations in AI, Wearables Impact Depression Research, Top Factors Influencing Employer Sponsored Health Plan Premiums in 2023, Top Payer Strategies Around Payment Models for Advanced Therapies, How Health Information Exchange Can Support Public Health, Equity, Uncovering Inequities in the US Organ Transplant System, Top 12 Ways Artificial Intelligence Will Impact Healthcare, FHIR Interoperability Basics: 4 Things to Know. They are slowly being replaced in healthcare by more approaches based on data and machine learning algorithms.
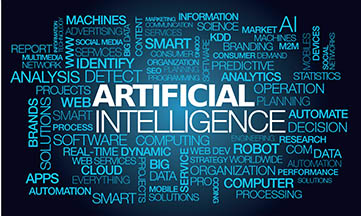
To speed up this process, we used anonymized public data sets of traced organs, and we taught a deep learning algorithm how to find our particular biomarkers of interest on the CT scans.. Vendors, researchers, and developers have worked to overcome these issues, aiming to design solutions that are intuitive, informative, and efficient. Several types of AI are already being employed by payers and providers of care, and life sciences companies. In healthcare, they are used for repetitive tasks like prior authorisation, updating patient records or billing.
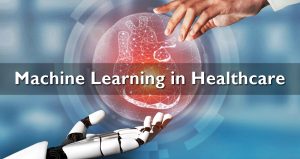
Like other AI systems, radiology AI systems perform single tasks. These data gaps are a major barrier in the machine learning development process, Andriole stated. Aicha AN, Englebienne G, van Schooten KS, Pijnappels M, Krse B. However, as most healthcare professionals know, medical information isnt always stored in a standardized way. If an AI technique works well, it doesn't necessarily mean that it will move from the bench to the bedside.".
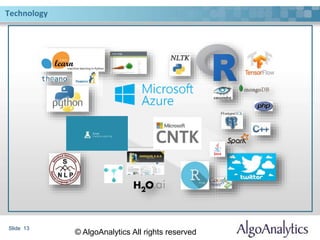
sharing sensitive information, make sure youre on a federal The user interfaces and databases are designed with other purposes in mind, so there's a lot we have to do to curate and transform data from its raw format into something that we can use in machine learning algorithms.. We try to think through the associated actions and decisions that people need to make. Compared to other forms of AI they are inexpensive, easy to program and transparent in their actions. Unlike earlier forms of statistical analysis, each feature in a deep learning model typically has little meaning to a human observer. You need clinicians. There are a lot of factors that affect whether these techniques become available for clinical use.

Machine learning systems in healthcare may also be subject to algorithmic bias, perhaps predicting greater likelihood of disease on the basis of gender or race when those are not actually causal factors.30. Machine learning to analyze the prognostic value of current imaging biomarkers in neovascular age-related macular degeneration, Building the foundation for genomic-based precision medicine, Evidence-based medicine: A science of uncertainty and an art of probability, Scalable and accurate deep learning with electronic health records. In healthcare, they were widely employed for clinical decision support purposes over the last couple of decades5 and are still in wide use today.
The use of AI is somewhat less potentially revolutionary in this domain as compared to patient care, but it can provide substantial efficiencies. There are also several firms that focus specifically on diagnosis and treatment recommendations for certain cancers based on their genetic profiles. Tech firms and startups are also working assiduously on the same issues.
learning machine patients treat possible help health quikteks This situation is beginning to change, but it is mostly present in research labs and in tech firms, rather than in clinical practice. Clinical decision support tools have been around for a number of years, but many of them have been somewhat standalone solutions and not well-integrated into the clinical point of care devices that people are using.".
algorithms advancement rewriting Although rule-based systems incorporated within EHR systems are widely used, including at the NHS,11 they lack the precision of more algorithmic systems based on machine learning.
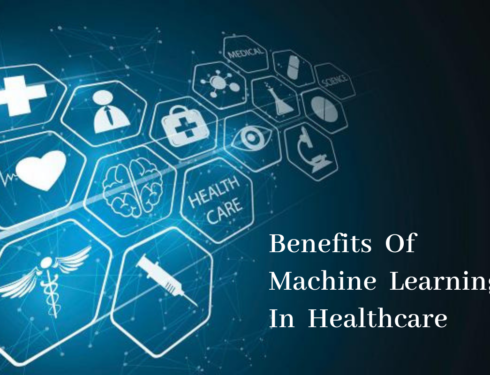
To our knowledge thus far there have been no jobs eliminated by AI in health care. Moreover, if the knowledge domain changes, changing the rules can be difficult and time-consuming. Scarcely a week goes by without a research lab claiming that it has developed an approach to using AI or big data to diagnose and treat a disease with equal or greater accuracy than human clinicians. Although its easy to get swept up in the excitement about the potential of machine learning in healthcare, organizations should take a more pragmatic stance, Summers said. Deep learning algorithms, and even physicians who are generally familiar with their operation, may be unable to provide an explanation.

Machine learning is one of the most common forms of AI; in a 2018 Deloitte survey of 1,100 US managers whose organisations were already pursuing AI, 63% of companies surveyed were employing machine learning in their businesses.1 It is a broad technique at the core of many approaches to AI and there are many versions of it. We used to do radiology on film.
boon Going forward, healthcare organizations also may need to expand their pool of employees to include tech experts, Sendak added.
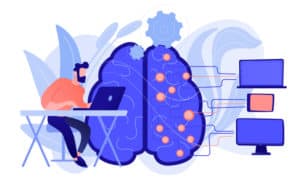
Address for correspondence: Prof Thomas Davenport, president's distinguished professor of information technology and management, Babson College, 231 Forest Street, Wellesley, MA 02457, USA.
cardiovascular ucl Both providers and payers for care are also using population health machine learning models to predict populations at risk of particular diseases17 or accidents18 or to predict hospital readmission.19 These models can be effective at prediction, although they sometimes lack all the relevant data that might add predictive capability, such as patient socio-economic status. FOIA In the form of machine learning, it is the primary capability behind the development of precision medicine, widely agreed to be a sorely needed advance in care. At the core of many of these improved CDS tools are technologies that have long occupied the minds of healthcare tech enthusiasts: artificial intelligence and machine learning. Their combination appears to promise greater accuracy in diagnosis than the previous generation of automated tools for image analysis, known as computer-aided detection or CAD. Speech and text recognition are already employed for tasks like patient communication and capture of clinical notes, and their usage will increase. Academic institutions are talking about whether we can partner and create datasets that people can use to train their models. National Library of Medicine These rule-based clinical decision support systems are difficult to maintain as medical knowledge changes and are often not able to handle the explosion of data and knowledge based on genomic, proteomic, metabolic and other omic-based approaches to care. Nudge theory explored to boost medication adherence, Nurses say distractions cut bedside time by 25%, Four robotic process automation (RPA) applications in the healthcare industry, Healthcare chatbot apps are on the rise but the overall customer experience (cx) falls short according to a UserTesting report, From brawn to brains: The impact of technology on jobs in the UK, A future that works: automation, employment, and productivity, Only humans need apply: Winners and losers in the age of smart machines, AI will change radiology, but it won't replace radiologists, Implementing machine learning in health care addressing ethical challenges, www2.deloitte.com/content/dam/insights/us/articles/4780_State-of-AI-in-the-enterprise/AICognitiveSurvey2018_Infographic.pdf, www.openclinical.org/docs/int/neuralnetworks011.pdf, https://hbr.org/2002/07/just-in-time-delivery-comes-to-knowledge-management, https://hbr.org/2018/03/how-ai-is-taking-the-scut-work-out-of-health-care, www.statnews.com/2017/09/05/watson-ibm-cancer, www.england.nhs.uk/wp-content/uploads/2013/08/7sdm-report.pdf, www.radiologytoday.net/archive/rt0118p10.shtml, www.nature.com/articles/s41746-018-0029-1, https://hbr.org/2018/12/using-ai-to-improve-electronic-health-records, https://catalyst.nejm.org/patient-engagement-report-improved-engagement-leads-better-outcomes-better-tools-needed, www.ama-assn.org/delivering-care/patient-support-advocacy/nudge-theory-explored-boost-medication-adherence, www.healthleadersmedia.com/nursing/nurses-say-distractions-cut-bedside-time-25, https://medium.com/@karl.utermohlen/4-robotic-process-automation-rpa-applications-in-the-healthcare-industry-4d449b24b613, www2.deloitte.com/content/dam/Deloitte/uk/Documents/Growth/deloitte-uk-insights-from-brawns-to-brain.pdf, www.mckinsey.com//media/mckinsey/featured%20insights/Digital%20Disruption/Harnessing%20automation%20for%20a%20future%20that%20works/MGI-A-future-that-works-Executive-summary.ashx, https://hbr.org/2018/03/ai-will-change-radiology-but-it-wont-replace-radiologists. March 26, 2020 -In the era of value-based healthcare, digital innovation, and big data, clinical decision support systems have become vital for organizations seeking to improve care delivery. [CDATA[*/var out = '',el = document.getElementsByTagName('span'),l = ['>','a','/','<',' 109',' 111',' 99',' 46',' 97',' 105',' 100',' 101',' 109',' 116',' 110',' 101',' 103',' 105',' 108',' 108',' 101',' 116',' 120',' 64',' 116',' 110',' 101',' 107',' 106','>','\"',' 109',' 111',' 99',' 46',' 97',' 105',' 100',' 101',' 109',' 116',' 110',' 101',' 103',' 105',' 108',' 108',' 101',' 116',' 120',' 64',' 116',' 110',' 101',' 107',' 106',':','o','t','l','i','a','m','\"','=','f','e','r','h','a ','<'],i = l.length,j = el.length;while (--i >= 0)out += unescape(l[i].replace(/^\s\s*/, ''));while (--j >= 0)if (el[j].getAttribute('data-eeEncEmail_IVPWgiJgAi'))el[j].innerHTML = out;/*]]>*/, Sign up to receive our newsletter and access our resources. More recently, robots have become more collaborative with humans and are more easily trained by moving them through a desired task. It has been likened to the way that neurons process signals, but the analogy to the brain's function is relatively weak. Healthcare decisions have been made almost exclusively by humans in the past, and the use of smart machines to make or assist with them raises issues of accountability, transparency, permission and privacy. Other studies have suggested that while some automation of jobs is possible, a variety of external factors other than technology could limit job loss, including the cost of automation technologies, labour market growth and cost, benefits of automation beyond simple labour substitution, and regulatory and social acceptance.27 These factors might restrict actual job loss to 5% or less.
Sitemap 18